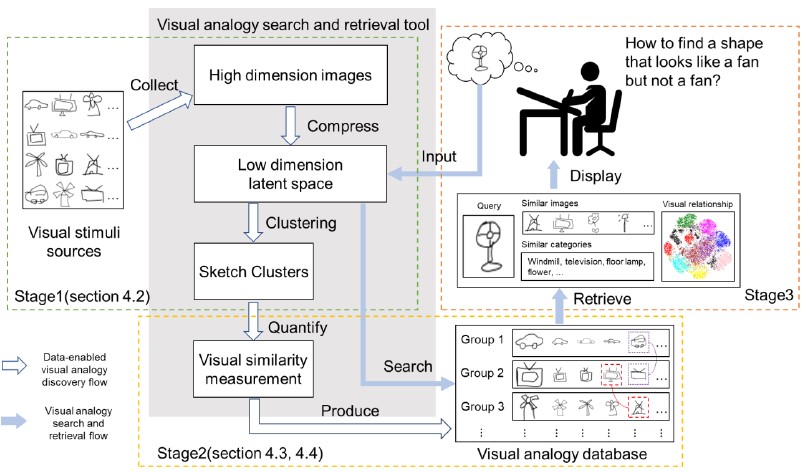
Graduate Researcher:
Zijian (Jamey) Zhang
Faculty Adviser:
Prof. Yan Jin
Visual analogy has been recognized as an important cognitive process in engineering design. Human free-hand sketches offer a useful data source for facilitating visual analogy. Although there has been research on the roles of sketching and the impact of visual analogy in design, little work has been done aiming to develop computational tools and methods to support visual analogy from sketches for design. Our goal is to develop a computer aided visual analogy support (CAVAS) framework that can provide relevant sketches from a variety of categories and stimulate the designer to make more and better visual analogies at the ideation stage of design.
The challenges of this research include what roles a computer tool should play in facilitating visual analogy for designers, what the relevant and meaningful visual analogies are at the sketching stage of design, and how the computer can generate such meaningful sketches from various categories through analyzing the sketches drawn by, or in response to, the designer. We propose a visual stimulation- based analogy support framework and a deep learning-based sketch generation and a deep clustering model to learn a latent space which can reveal the underlying shape features for multiple categories of sketches and at the same time cluster the sketches. The latent space learned serves as a statistical sketch generator that can present to the designer the relevant sketches, either automatically or in response to the designer’s request, together with the category information. The distance-based and overlap-based similarities are used to determine the long-distance and short-distance analogies and detect the bridging categories. Extensive evaluations of the performance of our proposed methods are carried out with different configurations. In addition, a visualization analysis of sketches in the latent space is provided to explore their visual similarities. The results have shown that the proposed methods can discover shape patterns embedded in different sketch categories and generate visual sketches for these categories to support designers’ visual analogy.
Related publications:
Zhang, Z. and Jin, Y. “An Unsupervised Deep Learning Model to Discover Visual Similarity between Sketches for Visual Analogy Support” IDETC2020-19700, Aug. 16-19, 2020, St. Luis, MO, USA
Zhang, Z, Gong, L., Jin, Y., Xie, J., Hao, J. “A quantitative approach to design alternative evaluation based on data-driven performance prediction”, International Journal of Advanced Engineering Informatics, 32, pp.52-65, Elsevier, 2017.